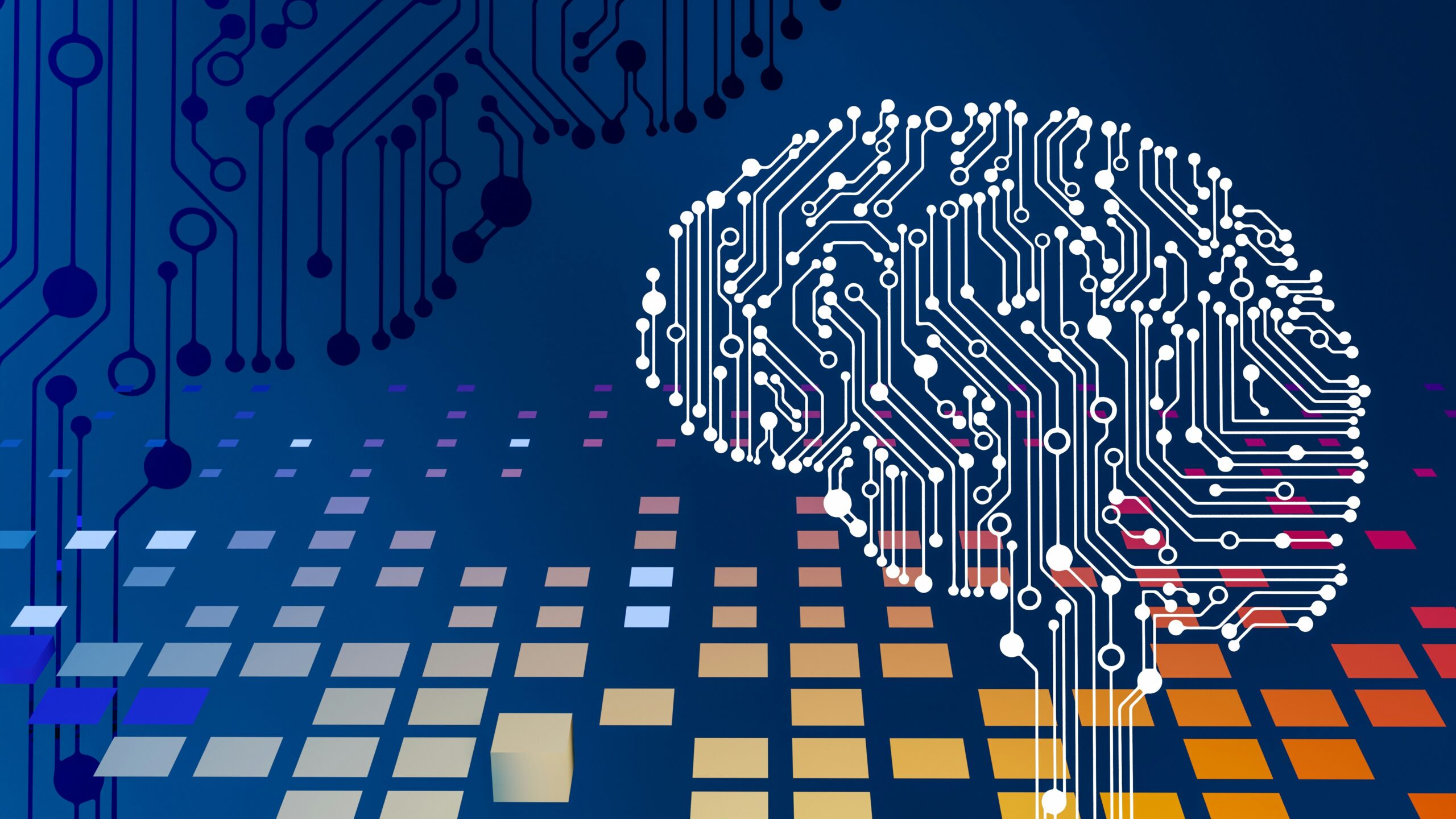
Getting Started with AI: A Simple Guide for Business
Why This Matters
Want to use AI in your business? It’s not as hard as you think. But you need a good plan. This guide will show you how to do it safely and smartly.
Why Take It Step by Step?
Starting with AI is like learning to drive. You don’t jump on the highway first. Here’s why taking it slow helps:
New Tech Takes Time
AI is new and complex. It’s like having a new powerful tool. You need time to learn how it works. Taking small steps helps you make better choices.
You Need the Right Skills
Think of AI like a new kitchen tool. Your team needs to learn how to use it. Some might need training. Others might need to join your team. Step by step, you build these skills.
Safety First
AI can be tricky. It’s like storing important data. You need to keep it safe. Taking time helps you spot problems before they get big.
Making It Fit Your Needs
Every business is different. You need to adjust AI to work for you. It’s like tailoring a suit. Small changes make it perfect.
Getting Everyone On Board
Different teams need to work together. IT, security, and business teams all play a part. Going step by step helps everyone understand their role.
Three Simple Steps to Start
1. Explore
First, play and learn:
- Try simple AI tools
- Work with different teams
- Start small and safe
- Write down what you learn
- Look for easy wins
2. Develop
Next, build something small:
- Pick one problem to solve
- Use ready-made AI tools
- Test everything carefully
- Ask teams what they think
- Make it better based on feedback
3. Deploy
Finally, put it to work:
- Start using AI in real work
- Look for new ways to use it
- Make a clear plan
- Keep everything secure
- Watch how it’s working
Quick Tips for Success
- Start with easy projects
- Learn from each step
- Keep security in mind
- Talk to your teams often
- Measure what works
Bottom Line
Taking small steps with AI helps you:
- Learn safely
- Avoid big mistakes
- Get better results
- Keep your data safe
- Help your team grow
Remember: You don’t need to rush. Each small step forward is progress. Start small, learn lots, and grow from there.
Want to Start?
Here’s what to do next:
- Pick a small project
- Get your team together
- Try some AI tools
- Learn what works
- Build from there
The key is to keep it simple and stay safe while you learn.
Comment (1)
A WordPress Commenter
Hi, this is a comment.
To get started with moderating, editing, and deleting comments, please visit the Comments screen in the dashboard.
Commenter avatars come from Gravatar.